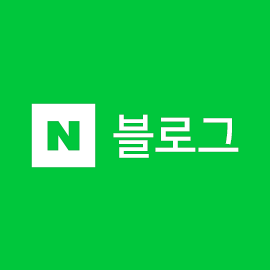
#Inceptionv4 #Classification >> 실험 결과에서 보았듯이, Residual connection은 현격하게 학습 속도를 높여줄 수는 있지만, Residual connection의 여부와 상관없이 성능은 결국 Model size의 문제(virtue of the increased model size)라고 결론을 내립니다. 이번에 소개할 논문은 2017년에 나온 Inception-v4, Inception-ResNet and the Impact of Residual Connections on Learning 입니다. 저자는 Szegedy 입니다. Inception-v1(GoogLeNet), Inception-v2, v3은 이미지 분류 대회에서 항상 좋은 성적을 거둬왔습니다. Inception 계열의 특징은 적은 파라미터수를 갖지만 모델이 다소 복잡합니다. 이 복잡성 때문에 VGGnet이 GoogLeNet보다 흔하게 사용되었죠. inceptio-v1에서 개선된 v4에는 ...
#Classification
#Inceptionv4
원문링크 : Inception-v4(2016)