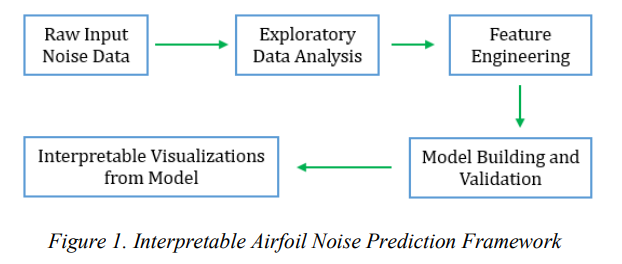
#Interpretability, #XGBOOST, #Random Forest, #Sound Pressure Level Prediction, #Wind Tunnel Speed , Angle of Attack, Airfoil, #Permutation Importance, Joint Feature Importance, #Swarm Plots, #Impact Analysis, #Shapely Additive Values and #Individual Conditional Expectation AI 또는 머신러닝에 대한 분석 결과를 이해하기 위해 블랙박스와도 같은 모델에 대한 이해가 선행되어야 한다. 이 논문에서는 시각화 분석 방법을 통해 NASA의 오픈소스 데이터셋을 활용하여 검증한다. dataset : 비행기 날개 단면 2, 3 차원 wind tunnel 분석 자료 (속도, 각도 등) 아래 두 가지의 기술적 접근 방법론 적용 1. Prediction of sound pressure lev...
#Impact
#설명
#가능
#XGBOOST
#xai
#Wind
#Swarm
#Sound
#Shapely
#Random
#Permutation
#Interpretability
#Individual
#인공지능
원문링크 : (논문 리뷰) Explainable and Visually Interpretable Machine Learning for Flight Sciences